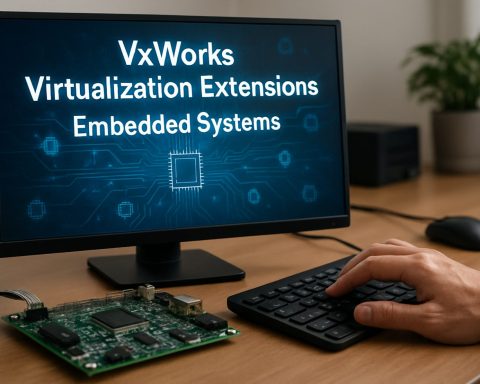
VxWorks Virtualization Extensions: Revolutionizing Embedded Systems in 2024
VxWorks Virtualization Extensions Unveiled: Transforming Embedded System Flexibility and Security for Next-Gen Applications. Discover How Wind River’s Latest Innovation Sets a New Standard. Breaking